

If the rectangular window were replaced by a smoothly decreasing (e.g. (The sinc function, you may recall, is the inverse Fourier transform of a rectangular window). The image produced by zero filling is equivalent to the convolution of the original image with a sinc function. Sampling low frequencies in k-space is equivalent to using a rectangular sampling window in the frequency domain. Zero-filling (ZIP) methods, commonly used in MRI, are responsible for truncation artifacts. Regardless of the technology used to capture the image, there are. Compare image adjustment modes and choose the best one for. These misleading appearances in pictures are generally called artifacts. Artifacts is the name I give to images of objects which were not originally designed to be Art, but which end up beautiful in some way, either because of some beauty in their design, or just because of a juxtaposition with other objects, or even just how the light hits them.
ARTIFACT IMAGES SERIES
This unusual feature occurs because the Fourier series is point-wise, but not uniformly convergent. The program restores images with different compression rates. He described the overshoot and ripples arising at sharp edges which we now appreciate as truncation artifacts on MR images.Īn interesting mathematical feature of the Gibbs phenomenon is that even with an infinite number of terms, the ripples will disappear but a remaining 8.9% overshoot will forever exist at the discontinuities.
ARTIFACT IMAGES FREE
(Note that if an apostrophe is used it must be placed after the "s", not before it - Gibbs', not Gibb's!)Īmongst his many other contributions to science (including Gibbs free energy in thermodynamics and the vector dot- and cross-product notations in math), Gibbs studied how Fourier series behaved at discontinuities. The Gibbs (truncation) artifact is named after 19th Century mathematical physicist Josiah W. The proposed method could easily be adapted to estimate a motion severity score, which could be used as a score of quality control or as a nuisance covariate in subsequent statistical analyses.Additional Notes on the Gibbs Artifact and Phenomenon Our results suggest that the proposed CNN framework enables the network to generalize well to both unseen simulated motion artifacts as well as real motion artifact-affected data. The CNN displayed significantly larger TCT compared to the uncorrected images whereas the adversarial network, while improved did not show a significantly increased TCT, which may be explained also by its over-enhancement of edges.
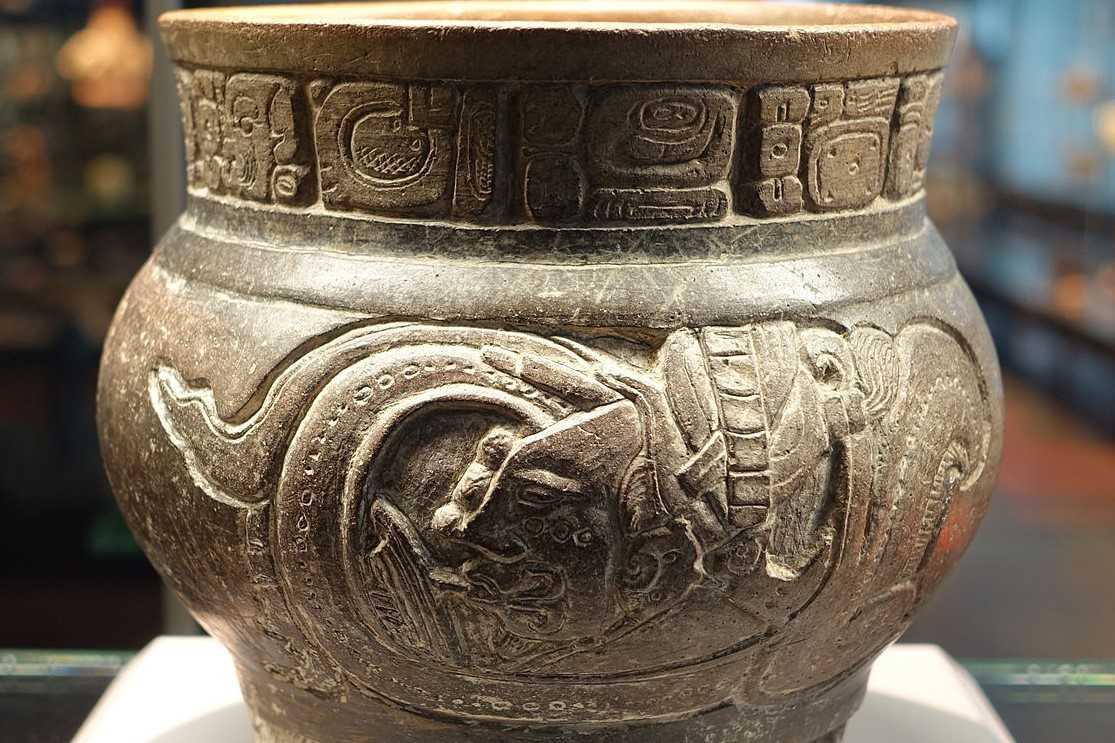
On the other hand, the two CNN models successfully removed the motion-related artifact as their SSIM and CC significantly increased after their correction and the error was reduced. It was found that Gaussian smoothing as a conventional method did not significantly differ in SSIM, CC and RMSE from the uncorrected data. Quantitative evaluation was carried out using metrics such as Structural Similarity (SSIM) index, Correlation Coefficient (CC), and Tissue Contrast T-score (TCT). Echogenicity is defined as the intensity of reflected sound waves.

The ultrasound image misrepresents the echogenicity of structures. The ultrasound image does not display existing structures. We tested the CNN on unseen simulated data as well as real motion affected data. The following artifacts are common in ultrasound imaging: The ultrasound image displays non-existing structures. We then added simulated motion artifacts on these images to produce motion corrupted data and a 3D regression CNN was trained to predict the motion-free volume as the output. For training, in order to gain access to a ground-truth, we first selected a large number of motion-free images from the ABIDE dataset. In this work, we propose to use convolutional neural networks (CNNs) to correct motion-corrupted images as well as investigate a possible improvement by augmenting L1 loss with adversarial loss. Abstract: Head motion during MRI acquisition presents significant problems for subsequent neuroimaging analyses.
